
When configuring the Airflow Kubernetes Executor, it is necessary to use the ‘template’, which is used to create new pods for subsequent tapes. A mistake in one task does not affect the other tasks. The advantage of this is that each task has its own dedicated resource space to use. In order for the Celery Executor to work properly, it is necessary to implement a message broker (RabbitMQ / Redis), which makes the configuration complicated.Īnother Executor supporting the work with a large number of tasks is the Kubernetes Executor, which runs each instance of the task in its own Kubernetes pod. This means that even if no task is being performed, resource costs are charged constantly. The limitation is that the number of workers and their resources must be defined in advance, and they are running all the time. These tasks can be performed in parallel on each worker, and the maximum number of tasks that one worker can perform is defined by the worker_concurency variable. The number of workers and their resources can be defined in advance. The first is the Celery Executor, which allows you to distribute tasks over many workers.
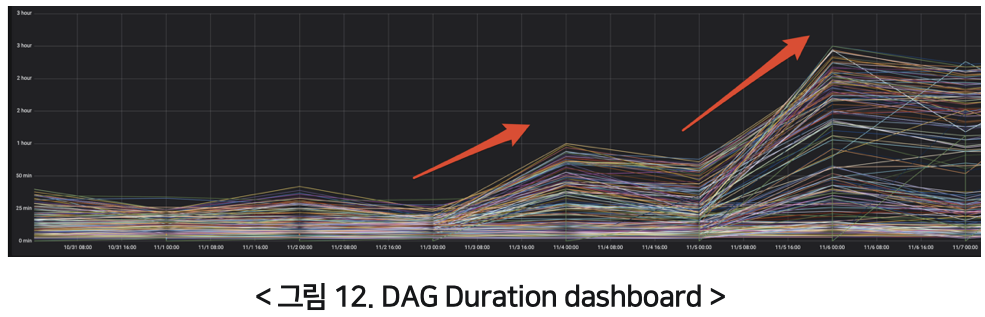
The Airflow Kubernetes Executor vs The Celery Executor – a first lookĪirflow has two executors in its resources which enable the parallel operation of many tasks.
#Airflow kubernetes worker how to
Would you like to learn how to configure it? Read our article to find out.
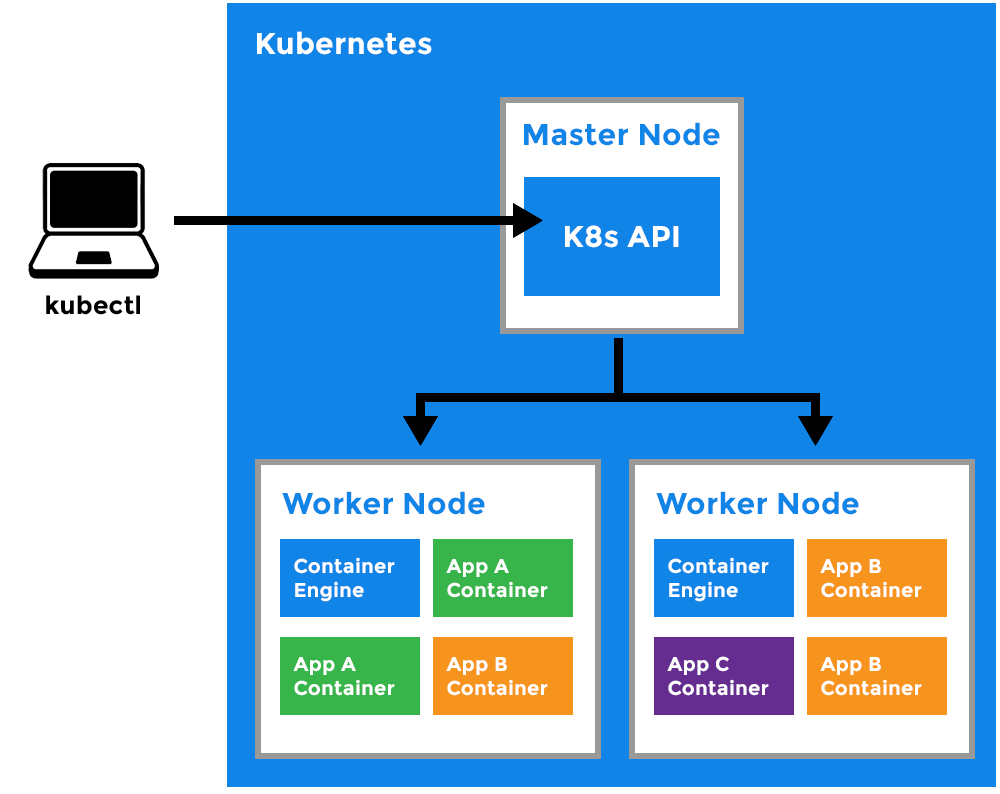
Have you got a dilemma because you don’t know which Executor to choose for your next Airflow project? The Celery Executor and the Kubernetes Executor make quite a combination – the Celery Kubernetes Executor provides users with the benefits of both solutions.
